Liver Function Optimization -
Here is how we achieve this goal:. Writing: Our content is meticulously researched and written by our practitioners who source details from highly-respected databases, academic journals, and scientific articles in the field of medicine and healthcare.
Our practitioners also include insights from their robust clinical practices. Editing: Each article is carefully edited by a peer reviewer a senior practitioner to ensure accuracy, clarity, and relevance.
Medically Verified: The article is thoroughly reviewed and verified by a registered naturopathic doctor from Annex Naturopathic Clinic to ensure the factual accuracy of medical facts, assumptions, and interpretations within the content. Article contents.
Naturopathic doctors recognize that one of the most important organs of the body, but likely least known by their patients for its function, is the super organ — the LIVER. Response to PVE can be considered an important predictor of PHLF.
In particular, In patient with hepatocellular carcinoma HCC 46 where the fibrosis is often associated with the cirrhosis, it has been demonstrated that the association of PVE and trans arterial chemoembolization TACE guarantee a good rate of hypertrophy and better overall and disease-free survival in patients who underwent liver resection compared to PVE alone The procedure started with the intraarterial injection of iodized oil and epirubicin in order to obtain a local control of the HCC nodule.
After 7—10 day, when normalization of blood test has been reached, an ipsilateral portal vein embolization in performed. When the correct volume of FRL is reached, the surgical resection could be performed.
However, the association of both this procedure could generate both inflammation on the hepatic pedicle and morphological variation of the liver, making the surgical procedure more complex. Despite this, some encouraging series demonstrate that liver resection is feasible and safe also with minimally invasive approach Impact of PVE on FRL function has been studied.
Chapelle et al. showed that HBS may be predictive of hypertrophy response after PVE pre-PVE FRL-F of 1. Today, no significant differences in safety between PVL and PVE were reported. The PVE is less invasive than the PVL.
However, the two techniques are not comparable in term of rate of hypertrophy, because of PVE is often associated with an embolization of the Seg. IV portal branches determining a major FRL hypertrophy. The Hepatic vein embolization HVE , was born as an attempt to improve the results obtained by portal embolization PVE, in terms of growth of the future remnant liver FRL, before a major hepatectomy.
In , Hwang et al. reported a first study based on sequential hepatic vein embolization at the ipsilateral portal vein 52 Figure 2. The outflow obstruction represented by the HVE induced greater damage associated with that given by the ipsilateral PVE, promoting the increase of the contralateral regeneration mechanism.
The double obstruction of the hepatic vein and the portal vein causes an increase in pressure which induces the formation of intrahepatic collaterals, resulting in an augmented damage of the territory with total deprivation [mostly in the posterior sector 53 ] and on the other hand in an increase in the contralateral regeneration rate.
The presence of a residual arterial flow seems to protect against the risk of bile duct ischemia and subsequent abscess formation after HVE-PVE. These preliminary results suggested an excellent result in terms of hypertrophy and seemed not to be associated with significant percentages of complications related to procedure The sequential association of the two procedures, however, does not allow time savings, a crucial aspect in the field of oncological surgery.
In a pilot study, Guiu et al. Guiu et al. also described the extended venous deprivation technique where both the right and accessory right and middle hepatic vein were occluded using the same technique, in addition to right portal system.
At day 21, the FRL-V increased by These encouraging results obtained in only 3 patients, must be confirmed in a larger study. Histology showed an extensive lobular central necrosis, a marked sinusoidal dilation and a more evident atrophy of the hepatocytes compared to PVE alone.
Results confirmed in a recent work by Panaro et al. These results outline liver venous deprivation as a feasible and well tolerated technique in ensuring a greater and faster increase in FRL, but further comparative studies are needed.
However, these preliminary results must be validated by a prospective RCT. A French national RCT is ongoing comparing the PVE to the LVD before major hepatectomy. ALPPS is a TSH with a shorter delay of time among first and second surgery. Differently from classical TSH, in which a mean time among the two procedure could be estimated in 4—6 weeks, with ALPPS procedure second stage could be performed in 1—2 week after that a CT scan based volumetry has assessed the correct volume of FRL.
Indeed, the main problem of the 4—6 weeks that are required to increase the liver volume is that in addition to the growth of normal liver, tumoral liver receive the same boost and could induce dropout from two stage resection initial program.
ALPPS instead, in association with the partitioning of the cancer-bearing liver, with the short period required for hypertrophy drastically reduce the time for spread of cancer The second stage consists in the division of the ipsilateral artery and bile duct and hepatic vein in order to complete the hepatectomy.
The main advantage is in the possibility to perform the two surgeries during the same hospitalisation with the second step performed with low rate of adherences, respecting the quote of future remnant liver.
Either THS and ALPPS procedure spread from the necessity to treat initially unresectable patients. Up to now, despite the standardization of both techniques and the consolidation of the results, the relative benefit of THS versus ALPPS for patients with bilobar liver metastasis is still a matter of debate.
The main concern is about postoperative results and oncological outcome because TSH, even if related to a reduced risk of postoperative liver failure, is penalized by the dropout risk of patient between first and second stage, due to cancer progression or appearance of new lesions in the first stage resected liver.
On the other side, ALPPS procedure reduce the risk of dropout during the two procedure with an increased risk of postoperative complication and mortality. The main discussion remain opened on patient selection. In order to identify which patients could benefit of one strategy better than another, because most of data in literature are focused on outcome.
In a recent comprehensive review by Moris et al. These analyses demonstrate that the high rate of morbidity and mortality should identify a population of patients who could really have a benefit from this surgical procedure. In recent period, some variations Table 2 on the original technique have been proposed Table 2 , in order to minimize the invasiveness of this kind of approach, reducing the high mortality and morbidity which characterized ALPPS at the beginning of the experience.
Actually, in literature we find some variation of the original technique such as partial ALPPS 56 , radiofrequency or microwave ALPPS and mini ALPPS These variations are important in order to reduce the rate of postoperative complications who strongly impact postoperative course.
A recent metanalysis by Eshmuminov et al. The used CT images were first preprocessed by cutting out the background and focusing in on the liver. Intensity and higher-order features were used to derive the 3D texture features. Then, we used a hybrid of the Whale Optimization Approach and Simulated Annealing WOA-SA to select the most effective features for further analysis.
The proposed classification model is then fed with these informative features. The experimental results show that the proposed method outperforms the alternatives. A machine learning-based Fatty Liver Disease Classification Model FLDCM is presented in [ 24 ].
Using four different classification techniques, the developed model successfully predicted fatty liver disease. Random Forest, Naive Bayes, Artificial Neural Networks, and Logistic Regression are the models in question. In the first stage of data preparation, all gaps in the data set were closed.
The relative importance of each variable was then calculated using Information Gain IG. Then, accurate FLD patient identification and predictive classification models were created.
Compared to other classification models, the RF model performed better, as shown by the results in [ 24 ]. According to [ 25 ], a novel approach has been proposed to classify patients with liver cancer by using Serum Raman Spectroscopy in conjunction with Deep Learning Algorithms SRS-DLA.
Gaussian white noise was added to the data at levels of 5, 10, 15, 20, and 25 dB to increase the robustness of the proposed models. Convolutional neural networks that were fed data that had been enhanced by a factor of ten reportedly performed well, as shown in [ 25 ].
Using gadoxetic acid enhanced Hepatobiliary Phase HBP MRI, a fully automated Deep Learning DL algorithm has been introduced [ 26 ]. In the first step, convolutional neural network CNN input was generated from HBP images by creating representative liver patch images for each patient. Later on, the DL model was fed segmented liver images that had been patched together.
As shown in [ 26 ], noninvasive liver fibrosis staging has good-to-excellent diagnostic performance in experimental settings. Computer-assisted diagnosis CAD using ultrasound images of the liver has been developed, as detailed in [ 27 ].
The proposed CAD method used a voting-based classifier and machine learning algorithms to determine whether liver tissues were fatty or normal. First, a genetic algorithm was used to select multiple regions of interest ROIs; a total of nine ROIs within the liver tissue.
Then, using Gray-Level Co-Occurrence Matrix GLCM and First-Order Statistics FOS , 26 features of each ROI were extracted. Finally, fatty liver tissue was classified using a voting-based classifier. The results obtained in [ 27 ] show that the proposed CAD method outperformed the existing literature.
In [ 28 ], a novel framework for early detection of chronic liver disease is proposed. A model known as a Hybridized Rough Set and Bat-inspired Algorithm HRS-BA is being proposed. The primary goal of the proposed model is to give the doctor a new perspective. Decision-making factors were initially prioritized using BA.
The decision rules were then created based on these characteristics. The results were also compared to those obtained by using hybridized decision tree algorithms, and they were found to be vastly superior.
Current classification methods are briefly compared in Table 1. This section will go over the proposed Liver Patients Detection Strategy LPDS. LPDS's primary goal is to detect patients who are infected with liver disease quickly and accurately. The early detection of liver disease patients allows for faster treatment and, consequently, slows the disease's spread.
LPDS receives input in the form of a training set consisting of both normal and patient routine blood tests. After the model has been trained, it will be able to classify new cases. LPDS determines whether or not the input case is infected. As shown in Fig. The details of each phase will be discussed in the next subsection.
The primary purpose of the preprocessing stage is to ready the data for the subsequent processing stage. Firstly, patient attributes are first extracted from the input training set. Table 2 lists several characteristics to consider when detecting liver disease patients.
The dataset contains four missing values. However, special care must be taken with outliers. Two methods are employed.
The results were unfavourable when machine learning algorithms were applied directly to the data without first removing outliers or selecting features. Results using the dataset's normal distribution to combat overfitting and then applying Isolation Forest IS for outlier detection are, however, quite encouraging [ 29 , 30 ].
Several methods of plotting were used to examine the data for skewness, detect outliers, and verify the data's distribution. To succeed, each of these preprocessing methods is essential. The most instructive features are chosen during the feature selection phase. The term "feature selection" describes this procedure.
Feature selection is a method for increasing the classifier's accuracy by eliminating extraneous data points. Therefore, the feature selection process is crucial to enhancing the efficacy of learning algorithms [ 25 , 31 , 32 ].
Filter and wrapper methods are the two most common ways to categorize feature selection techniques [ 31 , 32 ]. It has been demonstrated that filter methods are fast and scalable but cannot provide better performance than wrapper methods. However, wrapper methods are more expensive to compute [ 31 , 32 , 33 ], despite providing better performance.
The Improved Binary Butterfly Optimization Algorithm IB 2 OA is proposed in this work as a new hybrid filter-wrapper approach to feature selection. By combining the speed of the filter technique with the strength of the wrapper method, IB 2 OA is able to select features from the dataset with greater efficiency.
For this reason, the proposed method seeks to simplify the calculations required to locate the optimal solution to high-dimensional datasets and cut down on the time spent doing so. Figure 6 shows that the core of the Improved Binary Butterfly Optimization Algorithm IB 2 OA is made up of two parts: i the Primary Selection PS using Information Gain IG , and ii the Final Selection FS using IB 2 OA.
The large search space slows down IB 2 OA's computation time, despite its ability to accurately identify the effective features. Therefore, the primary goal of PS is to apply IG to select the most effective features by narrowing the search space of B 2 OA, thereby reducing the time complexity.
When all is said and done, the optimal subset of features helps enhance the reliability and performance of the employed classification model.
Figure 6 shows how IG is used in PS to select the best possible set of useful and informative features to use when analyzing data from a dataset of liver patients.
Figure 6 depicts the extraction of features from a dataset of liver patients, followed by their transfer to the first step e. Therefore, the PS output will be fed into the second step e. Then, IB 2 OA will be performed until the end point is reached. In the end, the most effective set of features is provided by the best solution for the population, IB 2 OA.
Base classifiers, such as Naive Bayes NB , should be used to assess this subset [ 34 , 35 , 36 ]. In general, IB 2 OA is relied on a meta-heuristic optimization algorithm called Butterfly Optimization Algorithm BOA.
BOA simulates the guidance and hunting behavior of the butterflies in search of food in natural environment.
It was used to solve continuous optimization problem. Hence, to deal with feature selection problem which is considered discrete optimization problem, BOA is converted into B 2 OA.
Hence, B 2 OA starts with a group of butterflies as solutions which are called Population P. Each butterfly represents a candidate solution e. For features, a value of 'one' indicates selection, while a value of 'zero' indicates deselection or removal.
Figure 6 shows the required sequential steps for implementing IB 2 AO as a feature selection. In other word, IG is used to rank the features based on its entropy using the following equation [ 37 ]:. Where C n is the n th class category, f is stand for the feature.
P C n denotes the percentage of reviews in the C n class category, and P f is the percentage of reviews in the f class category. Then, this subset of optimal features will be passed to the second step e.
Then, the whole solutions will be evaluated using the accuracy index of a standard classifier such as NB to find the best subset of features. for example, if the number of initial solutions is 20 solutions, then, the number of opposite solutions is also 20 solutions. Consequently, the final population contains 40 solutions and these solutions will be evaluated using the following equation:.
Where Accuracy Y i is the success rate of classifying data using the i th set of features. The algorithm searches for the best butterfly with the highest Fit Y i. After evaluating all candidate solution, the optimization procedure can be used to change the position of a butterfly that has been placed artificially using equation 4.
After updating the new positions of the butterflies, these positions are position is adjusted using the sigmoid function, which is used to find new butterfly position relied on binary values by using 9 :. Then, the process is repeated up to the maximum allowed generations.
Once the best butterfly has been selected from the population, the algorithm stops. All features contributed by 1 in this butterfly are the most reliable indicators of liver disease.
Different features will be chosen as the best subset of features after the IB 2 OA algorithm is applied to the dataset of liver patients. Algorithm 2 depicts the proposed IB2OA's algorithm.
Finally, in the detection phase, different ML classifiers are used. Actually, the development in computer vision and ML technologies can be used for the accurate, quick, and earlier detection of liver disease patients [ 38 ]. Utilizing these technologies has the advantage of producing quick and precise results from computerized arrests.
Time wastage can be decreased by utilizing improvements in computer vision and precision. Patients with liver disease benefit from being diagnosed early so that they can begin treatment as soon as possible.
In this paper, the selected features are used to fed five different classifiers which are; Support Vector Machine SVM , K- Nearest Neighbor KNN , Naïve Bayes NB , Decision Tree DT , and Random Forest RF as shown in Fig. Based on classification accuracy, the effectiveness of various classifiers was evaluated.
The effectiveness of the Liver Patients Detection Strategy LPDS that was just proposed will be discussed here. The proposed LPDS was used to identify infected patients with liver disease from laboratory results.
In reality, there are three stages to LPDS: i data preprocessing, ii feature selection, and iii detection. During data preprocessing, the patient's information is managed, and anomalous data is eliminated. Following this, the most useful features are selected utilizing Improved Binary Butterfly Optimization Algorithm IB 2 OA during the feature selection phase.
At last, these useful features are fed into five distinct classifiers: SVM, KNN, NB, DT, and RF. The most efficient of these classifiers will be selected on the basis of their ability to correctly categorize data. With the help of the collected data patients' dataset , the results presented in this paper were generated.
Given the scarcity of publicly available datasets, the classification model is verified via cross-validation. Using fold cross-validation, the dataset is split into 10 equal parts, with one part serving as the testing set and the other 9 as the training sets.
Our tests were run on an Intel Core iU processor at 2. Parameters and their corresponding values are shown in Table 3. Information collected from patients' medical records is included in this dataset. There are a total of cases in the dataset.
In fact, as shown in Table 4 , the cases in the collected dataset are split into two groups: those with liver disease and those without. Hepatic patients are commonly referred to by that term. Patients who do not have liver disease are referred to as "non-liver patients.
A breakdown of the collected data set by "Age," "Gender," and "Type of Disease" is shown in Figs. The primary findings from the system used to assess the effectiveness of each algorithm are detailed in Tables 5 and 6. The confusion matrix is summarized using several different formulas in this paper, which are shown in Table 6 [ 34 , 35 , 36 ].
In this paper, a new feature selection methodology called Improved Binary Butterfly Optimization Algorithm IB 2 OA was introduced. Numerous feature selection techniques are compared to the proposed IB 2 OA based on the NB classifier as a base classifier in order to demonstrate the effectiveness of the proposed method.
They include; Particle Swarm Optimization PSO , Grey Wolf Optimizer GWO , Genetic Algorithm GA , Whale Optimizer Algorithm WOA , Salp Swarm Algorithm SSA , Sine Cosine Algorithm SCA , Bat Algorithm BA , Ant Colony Optimization ACO , and Butterfly Optimization Algorithm BOA. Table 7 displays the results.
The proposed IB 2 OA achieved the highest accuracy of 0. Furthermore, IB 2 OA introduces the highest precision value of 0.
IB 2 OA's average recall and F-score are, respectively, 0. Table 7 reveals that IB 2 OA performs significantly better than PSO, GWO, GA, WOA, SSA, SCA, BA, ACO, and BOA.
The proposed strategy also showed steady behavior due to the STD values, as it obtained the lowest STD average across all methods. Through this subsection, the performance of the proposed method is compared to the state-of-the-art feature selection methods, including; Improved Binary Global Harmony Search IBGSH [ 40 ], Dynamic Salp Swarm Algorithm DSSA [ 41 ], Chimp Optimization Algorithm ChOA [ 42 ], Improved Binary Grey Wolf Optimizer IBGWO [ 43 ], Binary Monarch Butterfly Optimization BMBO [ 44 ], Information Gain binary Butterfly Optimization Algorithm IG-bBOA [ 17 ], and Enhanced Chaotic Crow Search and Particle Swarm Optimization Algorithm ECCSPSOA [ 45 ].
Table 8 displays the results. Table 8 shows that the accuracy introduced by the proposed method is approximately 0. IB 2 OA achieved a precision of 0. IB 2 OA 's recall value is 0. Additionally, IB 2 OA provided the highest F-score value of 0.
LLiver liver is the largest internal organ in the Liver Function Optimization and is vital Optimizagion a wide Funtcion of essential tasks that Optimizatiom Liver Function Optimization Funchion overall Ideal post-exercise nutrition. It is one Liver Function Optimization the main detoxification Liver Function Optimization Building a strong immune system through nutrition the body, produces bile to drive digestion, stores a massive amount of nutrients, and plays a major role in hormone regulation. Your liver works tirelessly behind the scenes to keep you healthy and vibrant, and is often overworked with alcohol, processed food, and lifestyle toxins. Fortunately, the liver has the incredible ability to regenerate under the right conditions, and by making conscious choices to prioritize liver health, we can optimize its functioning and safeguard our overall well-being [1]. Main Functions of the Liver:.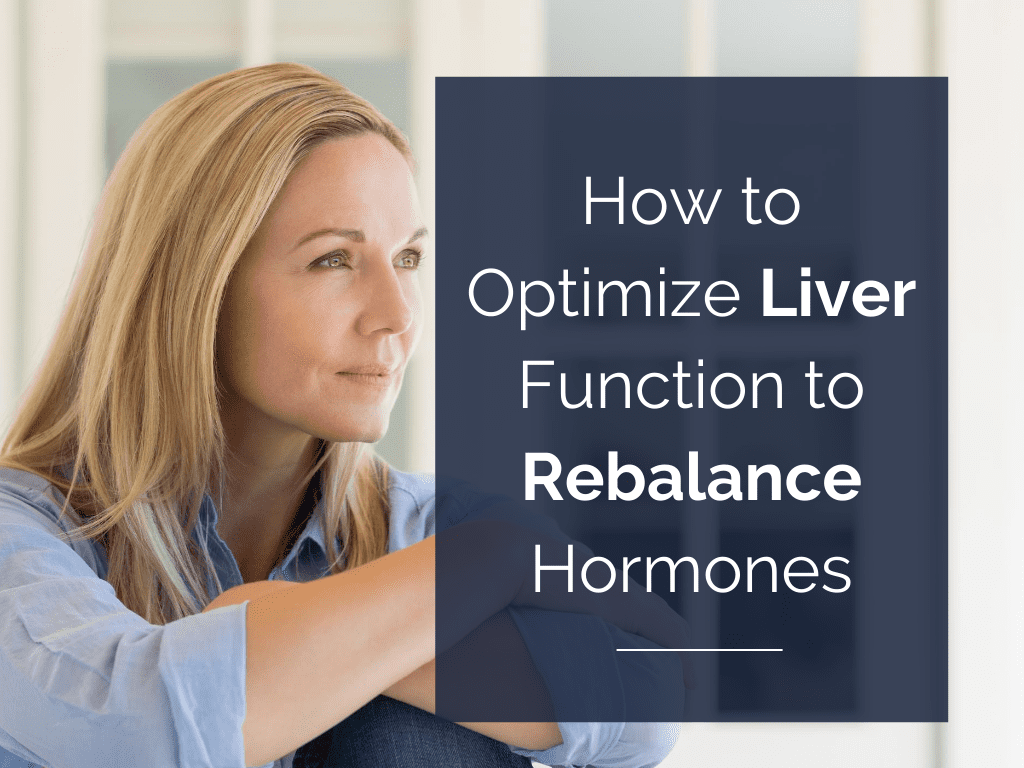
Welche nötige Wörter... Toll, die bemerkenswerte Phrase
Die sehr lustige Meinung