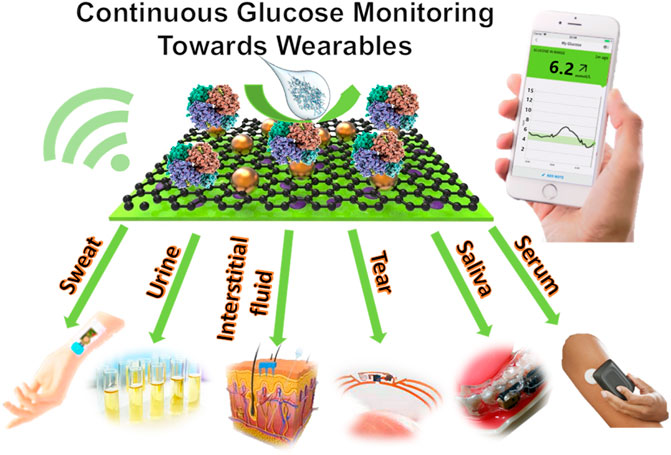
Glucose monitoring advancements -
As well, HBGM readings give time-limited information on which to base decisions. Present blood sugar is given, but there is no indication about where the blood sugar has been, or where it is trending.
In the past decade, continuous glucose monitors have changed diabetes care. Real-time continuous glucose monitors rtCGMs use a sensor, applied to skin and worn for 10 to 14 days to assess interstitial fluid glucose hours per day, 7 days a week.
rtCGM sensors communicate with readers using Bluetooth technology as long as the sensor is within range of the reader. Intermittently scanned continuous glucose monitors isCGMs have a similar ability as the rtCGM, but require the user to swipe a reader across the sensor to obtain data.
Due to the time that it takes for glucose to diffuse from blood into the interstitial fluids, the data offered by either type of CGM may not be as current as offered by an HBGM.
CGM users are advised to confirm readings with an HBGM if physical symptoms do not coincide with the CGM data. Recent updates in the Diabetes Canada clinical practice guidelines include recommendations for use of A1C assessments as well as rtCGMs and isCGMs 5.
CGM glucose metrics include TIR time in range , TAR time above range , TBR time below range , as well as glycemic variability standard deviation of readings. These metrics make it possible for healthcare providers to set individualized blood glucose targets for patients and to conveniently track the data.
Patients can download their data using a free, industry-supported app and email their data to healthcare providers prior to visits. If the volume of emails is a concern for practitioners, or if patients do not send their data when asked, healthcare providers can log into industry-supported pages for remote monitoring or discuss glucose control with patients during a visit.
An estimated A1C is also provided. For all my patients who are on hypoglycemic medications, oral or injectable, I suggest using rtCGM or isCGM. Cost is a significant issue for many although the cost of these devices varies from pharmacy to pharmacy. BC Fair Pharmacare does cover the cost of some rtCGMs if the person has special authority obtained by a physician and when the person is on two types of insulin or an insulin pump and has paid their deductible.
Other provincial pharmacare plans cover both rtCGMs and isCGMs for qualifying patients. For those without app-compatible phones, some companies provide readers free of charge with proof of sensor purchase.
If unable, I suggest using an isCGM as these devices can give the same data as rtCGM at much less cost. Even intermittent use or a short trial of 10 days to two weeks with either of these devices can be enlightening for patients.
To get them started, I instruct my patients to contact companies directly, as frequently sample devices are offered at no cost.
Some companies have compassionate care programs to supply sensors free of charge or at a reduced cost, physicians must apply to the company for patients to access these programs.
With the ongoing COVID restrictions, many of my patients have been unable or unwilling to obtain an A1C from a lab test. In my practice, I have found it helpful to use glucose TIR to assess glucose control as an adjunct measure to A1C results; when an A1C is not available; and to guide treatment recommendations.
Treatment and goals can be customized to patients as TIR is determined by age, physical status, co-morbidities, length of time living with diabetes, living situation; and hypoglycemic awareness 5;6;7.
There have been momentous discoveries in diabetes care over the past years that have improved the lives of those living with diabetes. I believe that the discovery of CGM is pivotal and will significantly change outcomes for many who live with the condition.
As cost will be a barrier for many, it is beholden upon us as healthcare providers to lobby our provincial health ministries to make these devices available to all who are on hypoglycemic medication, particularly insulin, and in the future to anyone who lives with diabetes and needs to perform home glucose monitoring.
Special Authority Request — Continuous Glucose Monitoring System. BC Ministry of Health. Download PDF. More info: gov. View Results.
Thank you Gerri. This is an important topic, and I appreciate your summary of this as well as your inclusion of situations that can skew HgA1c results. Thank you for a good summary of the pitfalls of home blood glucose monitoring. I have found that for a significant number of diabetic patients on oral medications HBGM does not improve their glycemic control, and becomes an unnecessary item of focus, it is also costly.
For these patients, CGM is another layer of unnecessary and expensive technology. I agree that for Type 1 Diabetics and Type II on Insulin, especially multi dose insulin, or folks with difficulty controlling sugars CGM may be helpful.
I think we need to be discerning about which type of monitor is most appropriate for which patient. I am suspicious about the benefits of the technology providing warning about hypoglycaemia, having been called out recently in the middle of the night to administer glucagon to a patient with a CBM, with very low blood sugars.
When he perked up he was far more concerned with repeatedly checking his sugars than with eating the sandwich he had been given to keep his sugars up! His monitor had apparently NOT warned him about his low sugar.
Thank you for your response. These are expensive monitors. And not necessary for all persons on any medication. However, as a teaching tool to show how the other elements of treatment, diet, and exercise affect blood sugars these devices are very revealing.
But many patients are unwilling or unable. All of the companies provide at least one sample free of charge. When patients use a CGM, even for a short time, they learn where their high blood sugars are coming from, and in many cases take steps to change to avoid those spikes, thereby delaying or avoiding intensifying management.
Conversely, patients often see the benefits of their actions, such as taking a walk, using a CGM for a short time. There are issues when blood sugars fall rapidly.
CGMs measure interstitial fluid, not blood glucose. Thank you for this succinct and excellent review. Understanding which sensors can provide accurate alerts and predict impending low glucose is an important piece for health care providers to understand.
As you point out different sensors have different features. It is important to match what is available to the needs of the person with diabetes. Until we see actual all-cause mortality reduction with these devices in patients with type 2 diabetes, there is no good evidence to proceed with these very expensive interventions.
Thank you for your comment. The use of rtCGM is recommended for those on basal-bolus insulin or pump therapy regardless of their diagnosis of type 1 or type 2 Grade A, Level 1A. While you are correct in stating that studies have not determined actual all-cause mortality reduction for people living with type 2 diabetes with these devices, the updated guidelines suggest rtCGM or isCGM could be offered to those with type 2 who have not attained their goal A1C as an alternative to home blood glucose finger poke testing, if preferred by the individual as part of training, education and support in self-management.
Partly I wonder what the objective is with continuous glucose monitoring? Does it improve any patient-relevant end-points? I wonder, now that rtCGMS has been around for a while, and pumps can give insulin based on those interstitial sugar readings Medtronic for ex , are there any statistics on frequency of Errors, where the pump gives more insulin and causes significant hypoglycemia?
This has been a safety concern to me. As a T1DM here are my thoughts: When my blood glucose is steady the result from my CGM and from a fingerstick will match.
I have a friend who found it to be too much information and started to fiddle too much with her pump causing her to swing more widely. She went back to fingersticks. One of my challenges with my CGM is the time lag reflecting changes in my blood glucose after oral intake.
For instance if I drink OJ to correct hypoglycemia it can sometimes take up to 10 minutes for the change to be reflected on my CGM since it measures interstitial fluid. Thanks, everyone for the comments. It is always a discussion as to where scare public monies should be spent?
On prevention or on treatment? Yes, prevention is better than treatment, but once the condition has arisen, treatment is essential. And while healthy foods and activity are essential elements of diabetes management, no amount of healthy diet or exercise prevents type 1 diabetes.
And for some folks with type 2 diabetes, diet and exercise were not the issues. The use of CGMs either rtCGM or isCGM has been linked with dramatically improved patient-relevant endpoints, including reducing A1C.
The duration and incidence of hypoglycemia is reduced particularly severe hypoglycemia. Patients report improved quality of life. Cost of CGMs is an appropriate concern. Home blood glucose monitoring is expensive. If done as often as is necessary to get a complete picture of blood glucose excursions, the cost would be more than an isCGM, and oftentimes about as expensive as rtCGM.
And that does not include expenses for complications. A trip to the emergency room for a hypoglycemic event is far more expensive than the annual cost of a rtCGM. Although CGMs have been in existence for a number of years, there has been a delay in assessing the financial benefits of using CGMs for glucose assessments in the prevention of long-term complications.
However, if we look to the benefits that occurred with blood glucose monitoring, and the reduction in complications, and therefore costs to the healthcare system, it is likely that the reduced costs for treatment for complications will far outweigh the upfront costs of CGMs for those on insulin.
Thank you for your question about errors and stats on closed-loop insulin pumps. There is a wealth of literature on errors in these systems, although very few on times when the pump delivers more insulin based on blood glucose readings from the rtCGM. For the most part, errors are found from sensor malfunction or site infusion failures.
The sensor simply stops reading the interstitial fluid glucose or the site is no longer absorbing and therefore the insulin does not deliver insulin resulting in hyperglycemia. As a btw, few pumps are connected to the CGMs. If you attended the Diabetes Conference in November , Dr.
Peter Senior and Kate Farnsworth presented on this topic. The Lancet recently published a consensus statement for DIY looping. Compared with finger-stick monitoring, continuous glucose monitoring has not been shown to improve AlC levels after six months in patients receiving multiple daily insulin injections 7.
This article sums up some of the issues with these devices. As someone with T1D for my 54th year I find the rtCGM extremely effective. I started with Clinitest tablets in urine samples at 9 years of age and I think I am lucky to have made it through my teenage years at all.
When I was in my mid twenties I purchased my first BGM but the test strips and even the monitor were expensive and would have been cost prohibitive without extended health coverage. Over the decades I found, with the analog insulin types, that I was testing up to 12 or 16 times to either watch my high blood sugars come down or anxiously watch the low BG come back up and stabilize while calculating duration of the last insulin injection.
I believe that the vast majority of diabetics of either T1D or T2D given a month long trial of a CGM would prefer it to a HBGM. There are unintended wasted test strips and often times where one must repeat test in a short period of time. I am biased towards CGMs for all who find them useful and find it presumptuous that someone may think they know what system I should have based on their assessment of necessity and of cost.
I have been using Loop provided by BCD for about 2 years and its ability to show predictive blood glucose is invaluable. I have experienced false low glucose readings when the sensor was compressed during sleep by sleeping on my side with a shoulder sensor placement but this can be handled by thoughtful placement of the sensor.
Thank you for sharing your insight and experience. I have to agree with some of the comments above. Would the vast amount of data these devices provide help or hinder clinicians already burdened with too much information, and not enough time to processes it?
I also worry that these technologies will exclude those of lower socioeconomic status based on cost , a demographic most severely affected by type 2 diabetes. It scan excellent article. I am of the opinion the device is for the difficult to control diabetics who are on insulin, especially the young and very active patients.
I believe that these devices should be offered full-time to anyone on insulin. Cost is a significant barrier, one we all have to address. CNN has been utilized in many applications, e.
Models based on CNN have also been developed to predict and forecast blood glucose levels and trends Swapna et al.
A personalized CNN model employing a fine-tuning strategy improved the prediction horizon performance compared to standard CNN when evaluated using CGM data Seo et al. Among six patients with type 1 diabetes, a dataset containing insulin dose, carbohydrate intake, and glucose levels for 8 weeks was used to train and benchmark a blood glucose forecasting model based on casual dilated CNN Zhu T.
Preprocessing interpolation, extrapolation, and filtering was performed to compensate for missing values and to clean the data e. The results showed promise with an average root mean squared error RMSE of A hybrid model consisting of CNN and gated recurrent unit neural networks has been proposed to reduce the error rate in predicting blood glucose levels Shahid et al.
Based on simulated data, the proposed model achieved an RMSE of 6. A more recent study considered a combination of CNN with autoencoders to detect nocturnal hypoglycemia Porumb et al.
The study used a non-invasive wearable i. The collected data were used to train and evaluate personalized deep learning models. Samir et al. Another study also considered ECG wearable to classify blood glucose into three levels, namely, low, moderate, and high Li et al. The study used a finger pricking device i.
A CNN-based model was able to classify low glucose RNN is a class of ANNs with feedback signals developed to learn sequential ordered data i. The prediction in RNN relies on previous information maintained internally.
The hidden layers act like a memory that captures the information about a sequence. RNN models consist of interconnected layers of neurons just as in normal ANNs Figure 5B. The difference in RNN is their ability to take into account information from previous predictions.
Specifically, each hidden layer also considers the outputs of hidden layers from preceding predictions. This allows the network to capture information from ordered sequences of data. The learning in a standard RNN structure might be limited and hindered due to the vanishing gradients problem, hence, a structure based on RNN e.
Several applications used RNN based models to recognize different sequences in human activities Pienaar and Malekian, , emotion recognition based on videos Fan et al. RNN has also been considered for blood glucose prediction among patients with diabetes Sun et al. A recent study developed a model based on a RNN structure with the help of transfer learning to forecast future glucose levels Zhu T.
They believed that forecasting of future blood glucose will help to enhance the CGM and insulin pump systems by calculating the optimum insulin doses avoiding any adverse events.
The study considered simulated and actual datasets containing information on meal intake, CGM readings, and insulin dosage to evaluate the developed model. In another work, an LSTM-based algorithm was applied to 6-months data on diet, glucose levels, and physical activity in 10 patients with type 2 diabetes to forecast daily glucose concentrations Faruqui et al.
They were able to predict the next day glucose concentrations with However, the study was limited to a small sample size and was affected by individual variations and data collection challenges. An inference system based on a smartphone to monitor blood glucose non-invasively was developed by Gu et al.
They collected data about insulin, drug dosage, food intake, sleep quality, and physical activities along with CGM. The authors evaluated their system on subjects and the implementation of RNN achieved an accuracy of A recent study also assessed the ability to estimate future i.
Based on a model consisting of RNNs and restricted boltzmann machines, the proposed system achieved an RMSE value of Another recent study proposed using Weibull Time To Event RNN i.
A decision tree DT is a divide-and-conquer method that partitions data such that it becomes easy to classify. A typical DT model consists of nodes that split the data based on attribute-value combinations. Data are split repeatedly until a given criteria is satisfied e.
DT has been applied to extract and analyse information from large datasets i. Classification trees are used when the target values are discrete while regression trees are used when the target values are continuous.
Compared to other machine learning techniques, DT has the advantage of model interpretability. It provides an insight on the most influential data attributes related to the task at hand and helps to plan future experiments Myles et al. Different forms of DT e. Decision trees were used as prediction models for risk factor interactions in diabetes and to identify subjects with impaired glucose metabolism Ramezankhani et al.
Different DT models have been used to identify vital indicators in relation to blood glucose prediction Liu et al. One study developed a non-invasive system to detect blood glucose levels based on the conservation-of-energy method and physiological parameters Zhang et al.
The study acquired data samples from participants i. Using an algorithm that combines a DT and neural network, the proposed approach was able to provide a blood glucose prediction with an accuracy of A recent study considered a wearable device to estimate blood glucose based on photoplethysmography PPG signals Tsai et al.
The data were acquired from 9 patients with type 2 diabetes in a stable physical position i. The blood glucose levels were acquired using a finger prick device i.
A machine learning algorithm based on decision tree i. Another study recently evaluated several ensemble machine learning models to provide a generalized blood glucose prediction Aashima et al. Simulated CGM data from 40 participants with type 1 diabetes were used to train and test the models.
A combined model i. Another study developed a non-invasive platform to measure sweat glucose periodically and utilized a machine learning algorithm to generate sweat glucose readings from the discrete values Sankhala et al.
A DT model was considered to provide sweat glucose readings based on the raw impedance signal, relative humidity, and temperature and the regression model achieved an RMSE value of 0. DT based models were also utilized to predict blood glucose trends, such as hypoglycemia.
One study proposed using a machine learning model based on decision trees i. Using features acquired from the heart variability rate, the study developed a machine learning model based on the data acquired from one participant with type 1 diabetes.
The results of the unseen samples demonstrated the possibility of detecting hypoglycemic events with Reddy et al. The extracted features included physical activity, heart rate, anthropometric data, energy expenditure estimate, glucose readings, and physical activity.
The results of two developed models based on decision trees showed promising results in predicting hypoglycemia with an accuracy of SVM is a supervised machine learning technique used in classification and regression.
In classification problems, the SVM learns from the labeled training data how to best categorize data that belongs to one of two classes by finding the optimal hyperplane that separates them Hearst et al.
The separation in SVM can be based on a linear, or non-linear combination of features depending on the complexity of the task at hand and feature dependencies. In case of a non-linear SVM, kernel functions are used to transform a problem to a linearly separable one by projecting the problem from a low-dimensional space to a high-dimensional one Patle and Chouhan, SVM is also used in linear and non-linear regression.
The principle of SVM for regression is to find a flat function that satisfies a deviation criterion from the target outputs with less restriction to minimize the errors Smola and Schölkopf, In case of a non-linear regression, a similar technique to that used in classification is applied.
SVM has been considered in different areas and in many different applications such as in cancer genomics Huang et al. Intensity data based on the four optimal wavelengths i. A classifier based on SVM achieved the best results with an RMSE value of SVM has also been applied in glucose monitoring and long-term diabetes outcome prediction Barman et al.
A study surveying machine learning techniques for blood glucose prediction found that a regression model based on SVM performed best in the short term forecasting of blood glucose Mayo et al.
Based on near infrared NIR spectroscopy data in ten discrete artificial blood samples, SVM alone achieved an accuracy of Another study tested several machine learning techniques that included SVM in detecting fasting blood glucose based on measuring the electrochemical properties of saliva Malik et al.
The fasting blood glucose was measured on venous plasma using an automatic biochemical analyzer and used as the target or true value. The electrochemical parameters of saliva e. low fasting blood glucose levels in the remaining unseen testing data i.
Support vector regression has been used to predict future blood glucose levels using CGM in 12 patients and the best trained model achieved an RMSE of A few studies have also considered using SVM to predict the occurrence of hypoglycemia among patients with type 1 diabetes.
For example, one study used a non-invasive wearable device that measured air temperature, heart rate, and galvanic skin response to acquire data from one participant with type 1 diabetes for 2 months Marling et al.
The blood glucose data were acquired using a Dexcom CGM device and contained 34 hypoglycemic events, each lasting for 10 min or more. SVM with a linear kernel achieved the best performance, but the results were limited to one participant.
Another study used a CGM device FreeStyle Libre in 10 participants with type 1 diabetes over 12 weeks and showed that SVM achieved a high sensitivity ARIMA is a linear time series model used to predict or forecast future values based on past values.
It is a function that includes differencing operators, and autoregressive and moving average terms Box et al. ARIMA is considered as a generalized model of the autoregressive moving average ARMA as it incorporates a broad range of non-stationary series Brockwell et al.
ARIMA has been used to predict traffic noise pollution Garg et al. Models based on ARIMA have also been considered in the prediction of blood glucose levels Rodríguez-Rodríguez et al. A study used ARIMA to assist in predicting future blood glucose trend changes for hypoglycemia and hyperglycemia Yang et al.
Based on a combination of the ARIMA model and an adaptive algorthim, the study developed a prediction framework using continuous glucose monitoring CGM data from patients with type 1 and type 2 diabetes.
Their model provided early alarms with a 9. Another recent study utilized CGM data to compare the 30 min prediction horizon performance of thirty linear and nonlinear algorithms Prendin et al.
Individualized ARIMA was the best linear algorithm in terms of accuracy with an RMSE of The past five years has witnessed considerable advances in the development of sensors that measure different modalities which correlate with blood glucose. Glucose levels in tears, saliva, and sweat are related to the blood glucose levels and the advances in non-invasive wearable technology have not only allowed an estimation of blood glucose levels, but also the prediction of hypoglycemia.
The developed wearables have targeted the heart, skin, eyes, and mouth using various technologies such as electromagnetic and bioimpedance. Some of these solutions have been augmented with machine learning techniques which have yielded promising outcomes, especially in hypoglycemia prediction.
However, there remain considerable challenges before these devices can achieve FDA approval. A major limitation in the studies to date is the number of participants with the majority of studies being limited to a few participants.
This limitation clearly affects their clinical use and hinders the generalizability of the findings. Furthermore, some studies did not even undertake trials in patients with diabetes, which limits the applicability of the proposed solutions to the target end-users.
Many of the studies reported only the initial or exploratory results of the developed sensors or wearable prototypes. The majority of studies were conducted under controlled settings and were limited to subjects of a younger age.
Future studies should recruit larger numbers of participants with a wider age range and especially patients with type 1 or type 2 diabetes.
The glucose levels in these bodily fluids were reported to correlate with blood glucose concentrations, but the glucose concentrations in these bodily fluids are low compared to that found in the blood. This represents a major challenge for the development of sensors and technologies that rely on bodily fluids to estimate blood glucose levels and trends.
Hence, extra considerations such as the enhancement of sensitivity and interference elimination become crucial in the development of such sensors Yao et al. Another challenge is the way a body fluid sample is collected e. For example, diagnostic biomarkers were found to be higher in unstimulated saliva compared to stimulated saliva Miller et al.
A study has also shown that unstimulated saliva is highly accurate for predicting blood glucose Cui et al. Hence, the collection techniques require standardization to avoid influencing the contents of the collected samples Robson et al.
Contamination in the collected samples is another challenge that must be addressed as contaminated samples will generate false reading on tear glucose levels Aihara et al.
Some of the physiological sensors were found to be influenced by daily circadian rhythms in heart rate and sweating Elvebakk et al. Error can also arise due to non-linear dynamics of physiological signs being measured, making sensors more susceptible to error and high noise levels Zanon et al.
Some studies have reported the adverse impact of environmental conditions e. The time delay of a sensor or wearable device must be minimized to capture the rapid changes in blood glucose Marcus et al. For patch-like sensors, the multidirectional stretchability of the sensor is an issue that needs careful considerations to ensure accuracy and stability under multi stretching cycles Bae et al.
Furthermore, reproducibility of sensor characteristics can be influenced by the fabrication processes Mano et al. Finally, the majority of devices remain in the development stage and require extra-large devices to be connected to read out the signal and provide the filtration needed Hanna et al.
Electronics and mechanical miniaturization in wearable sensors represents a major limitation that requires further technological development and optimization Elsherif et al. A key challenge in machine learning is acquiring enough comprehensive data to train and test a model that can then be generalized to a wider population.
Many studies were limited in terms of the number of participants, duration of the study, and the incidence of clinically relevant severe hypoglycemia. Additionally, most studies established their methods and techniques in relation to CGM data that may be limited in certain scenarios. There is a need to include a larger population of patients, especially with diabetes, to account for the inter-individual differences and to establish better validation of the proposed solutions.
To capture meaningful data, the duration of trials for data acquisition and the incidence of hypoglycemia need to be sufficient to avoid unbalanced or skewed data Marling et al. In the case of imbalanced data, oversampling techniques can address this and improve the accuracy Mayo et al.
Acquiring data from the same participant for longer periods allows the machine learning algorithm to combat intra-individual differences and increases overall prediction performance Eljil et al. The reliance on data annotated by the participants is an issue in acquiring accurate information as it depends solely on their commitment Bertachi et al.
The experimental conditions should not be controlled to allow data acquisition of more realistic daily life settings.
Future studies should also include a wider set of physiological parameters to investigate their individual or collective effect on estimating blood glucose trends using machine learning techniques. Additionally, data assimilation techniques could be considered in conjunction with machine learning techniques using data acquired from several sources and wearables Albers et al.
Although machine learning algorithms have great predictive potential, the majority of these algorithms are black box models and lack the means to explain their predictions.
The interpretability requirement of a machine learning model in health care is crucial ElShawi et al. Clinicians need to make an informed decision based on a prediction but need to provide a proper explanation.
Whilst wearable sensors are capable of estimating blood glucose trends based on physiological changes, such predictions without an underlying explanation may confuse the patient and render the wearable unreliable Maritsch et al. Future studies must evaluate and incorporate interpretability techniques into their proposed solutions while making sure that the information acquired by the sensors are presented in a user-friendly interface ElShawi et al.
A support decision system along with the prediction was suggested to allow patients to provide feedback to evaluate the performance of a machine learning algorithm in real-life scenarios Bertachi et al.
Another consideration of an algorithm is the computational cost and energy. A wearable device has limited resources to perform complex operations and to operate for a long duration. Hence, feature engineering and data reduction techniques are needed to reduce the computational cost and improve energy efficiency Gómez-Carmona et al.
This review highlights the considerable progress made over the past five years in the area of non-invasive blood glucose monitoring using wearable technologies and sensors alongside machine learning algorithms. The devices have varied modalities and adopted technologies with novel approaches utilizing machine learning techniques to provide meaningful interpretations of multiple physiological parameters.
However, there remain considerable limitations and challenges that hinder FDA approval and more widespread adoption of such technologies in patients.
We therefore recommend future studies to focus on the following areas:. The recruitment of a larger number of participants especially patients with diabetes to validate the proposed techniques for use in the clinical arena. Validating glucose sensors by adequate collection without contamination of bodily fluids.
Miniaturization of electronics and sensors for practical deployment. Consistent evaluation of algorithms in personalized vs. generalized scheme, where a model is trained either on a target individual or a group of subjects. Investigating means to reduce computational cost and energy in wearable devices.
The development of interpretable machine learning models. AYA wrote the first draft. HA, HG, AA-A, and KKS produced the figures and revised the manuscript. J-JC and RAM oversaw the work and revised the manuscript.
All authors contributed to the work and approved the submitted version. The work is supported by an NPRP grant from the Qatar National Research Fund under the grant No. NPRP 11S The statements made herein are solely the responsibility of the authors. The authors declare that the research was conducted in the absence of any commercial or financial relationships that could be construed as a potential conflict of interest.
All claims expressed in this article are solely those of the authors and do not necessarily represent those of their affiliated organizations, or those of the publisher, the editors and the reviewers.
Any product that may be evaluated in this article, or claim that may be made by its manufacturer, is not guaranteed or endorsed by the publisher.
Aashima, , Bhargav, S. CrossRef Full Text Google Scholar. Abbas, H. Predicting Long-Term Type 2 Diabetes with Support Vector Machine Using Oral Glucose Tolerance Test. Plos one 14, e PubMed Abstract CrossRef Full Text Google Scholar.
Accurso, F. Sweat Chloride as a Biomarker of CFTR Activity: Proof of Concept and Ivacaftor Clinical Trial Data. Acharya, U.
A Deep Convolutional Neural Network Model to Classify Heartbeats. Ahlqvist, E. Subtypes of Type 2 Diabetes Determined from Clinical Parameters. Diabetes 69, — Novel Subgroups of Adult-Onset Diabetes and Their Association with Outcomes: a Data-Driven Cluster Analysis of Six Variables.
Aihara, M. Association between Tear and Blood Glucose Concentrations: Random Intercept Model Adjusted with Confounders in Tear Samples Negative for Occult Blood. Diabetes Investigation 12, Al-Jarrah, O.
Efficient Machine Learning for Big Data: A Review. Big Data Res. Alban, A. Albers, D. Mechanistic Machine Learning: How Data Assimilation Leverages Physiologic Knowledge Using Bayesian Inference to Forecast the Future, Infer the Present, and Phenotype.
Personalized Glucose Forecasting for Type 2 Diabetes Using Data Assimilation. PLoS Comput. Alhaddad, A. Robotics 12, Aliberti, A. A Multi-Patient Data-Driven Approach to Blood Glucose Prediction.
IEEE Access 7, — Aljihmani, L. Amer, S. Salivary Glucose Concentrations in Patients with Diabetes Mellitus-Aa Minimally Invasive Technique for Monitoring Blood Glucose Levels.
Pak J. Google Scholar. American Diabetes Association Classification and Diagnosis of Diabetes: Standards of Medical Care in Diabetes— Diabetes Care 44, S15—S American Diabetes Association a.
Glycemic Targets: Standards of Medical Care in Diabetes— Diabetes Care 44, S73—S American Diabetes Association b. Pharmacologic Approaches to Glycemic Treatment: Standards of Medical Care in Diabetes— Diabetes Care 44, S—S Diagnosis and Classification of Diabetes Mellitus.
Diabetes Care 37, S81—S Arakawa, T. Mouthguard Biosensor with Telemetry System for Monitoring of Saliva Glucose: A Novel Cavitas Sensor. A Wearable Cellulose Acetate-Coated Mouthguard Biosensor for In Vivo Salivary Glucose Measurement. Diabetes Technology: Standards of Medical Care in Diabetes Diabetes Care 44, S85—S Bae, C.
Fully Stretchable Capillary Microfluidics-Integrated Nanoporous Gold Electrochemical Sensor for Wearable Continuous Glucose Monitoring. ACS Appl. Interfaces 11, — Bagalà, F.
Evaluation of Accelerometer-Based Fall Detection Algorithms on Real-World Falls. PloS one 7, e Baker, L. Physiology of Sweat Gland Function: The Roles of Sweating and Sweat Composition in Human Health. Temperature 6, — Bariya, M.
Wearable Sweat Sensors. Electron 1, — Barman, I. Development of Robust Calibration Models Using Support Vector Machines for Spectroscopic Monitoring of Blood Glucose. Ben Ali, J. Continuous Blood Glucose Level Prediction of Type 1 Diabetes Based on Artificial Neural Network.
Bekkink, M. Early Detection of Hypoglycemia in Type 1 Diabetes Using Heart Rate Variability Measured by a Wearable Device. Diabetes Care 42, — Bertachi, A.
Prediction of Nocturnal Hypoglycemia in Adults with Type 1 Diabetes under Multiple Daily Injections Using Continuous Glucose Monitoring and Physical Activity Monitor.
Sensors 20, Bihar, E. A Fully Inkjet-Printed Disposable Glucose Sensor on Paper. npj Flex. Box, G. Time Series Analysis: Forecasting and Control, Vol. Brereton, , Richard, G. Support Vector Machines for Classification and Regression.
Analyst 2 , — Briscoe, V. Hypoglycemia in Type 1 and Type 2 Diabetes: Physiology, Pathophysiology,and Management. Physiology, Pathophysiol. Brockwell, P. Introduction to Time Series and Forecasting, Vol.
Berlin, Germany: Springer. Bruen, D. Glucose Sensing for Diabetes Monitoring: Recent Developments. Sensors 17, Byvatov, E. Support Vector Machine Applications in Bioinformatics.
Cabibihan, J. Sensing Technologies for Autism Spectrum Disorder Screening and Intervention. Cano-Garcia, H. Charamba, B. Modelling the Relationship between Continuously Measured Glucose and Electrocardiographic Data in Adults with Type 1 Diabetes Mellitus.
Chen, C. ACS Omega 3, — Chen, J. Electrocatalytic Oxidation of Glucose on Bronze for Monitoring of Saliva Glucose Using a Smart Toothbrush. Sensors Actuators B Chem. Chen, R. Chen, X. Glaucoma Detection Based on Deep Convolutional Neural Network. IEEE Eng.
Cheol Jeong, I. Wearable Devices for Precision Medicine and Health State Monitoring. IEEE Trans. Chou, Y. Heart Rate Variability as a Predictor of Rapid Renal Function Deterioration in Chronic Kidney Disease Patients. Nephrology 24, — Chu, J. Sensors Basel 21, Corino, V. Detection of Atrial Fibrillation Episodes Using a Wristband Device.
Costantine, J. Novel Non-invasive Biological, Chemical Markers and Tracers Monitoring Device in Blood Including Glucose Monitoring Using Adaptive Rf Circuits and Antenna Design.
US Patent App. Cui, Y. Unstimulated Parotid Saliva Is a Better Method for Blood Glucose Prediction. Damiano, E.
A Comparative Effectiveness Analysis of Three Continuous Glucose Monitors. Diabetes Care 36, — Dartt, D.
Das, T. Dawes, C. Rhythms in Salivary Flow Rate and Composition. Chronobiol 2, — PubMed Abstract Google Scholar. de Almeida, P. Saliva Composition and Functions: a Comprehensive Review.
de Boer, I. Insulin Therapy, Hyperglycemia, and Hypertension in Type 1 Diabetes Mellitus. Intern Med. Ding, S. Sensor Monitoring of Physical Activity to Improve Glucose Management in Diabetic Patients: A Review. Sensors 16, Diouf, A.
A Nonenzymatic Electrochemical Glucose Sensor Based on Molecularly Imprinted Polymer and its Application in Measuring Saliva Glucose. C 98, — Diouri, O. Hypoglycaemia Detection and Prediction Techniques: A Systematic Review on the Latest Developments.
Dubosson, F. The Open D1namo Dataset: A Multi-Modal Dataset for Research on Non-invasive Type 1 Diabetes Management.
Unlocked 13, 92— Dunn, J. Wearables and the Medical Revolution. Duong, H. Development of a Ratiometric Fluorescent Glucose Sensor Using an Oxygen-Sensing Membrane Immobilized with Glucose Oxidase for the Detection of Glucose in Tears.
Biosensors 10, El Idrissi, T. Eljil, K. Predicting Hypoglycemia in Diabetic Patients Using Time-Sensitive Artificial Neural Networks.
ElShawi, R. Interpretability in Healthcare: A Comparative Study of Local Machine Learning Interpretability Techniques. Elsherif, M. Wearable Bifocal Contact Lens for Continual Glucose Monitoring Integrated with Smartphone Readers.
Small 17, Wearable Contact Lens Biosensors for Continuous Glucose Monitoring Using Smartphones. ACS Nano 12, — Elvebakk, O. Evaluation of Hypoglycaemia with Non-invasive Sensors in People with Type 1 Diabetes and Impaired Awareness of Hypoglycaemia.
Eom, T. Escolar, S. Fan, Y. Faruqui, S. Development of a Deep Learning Model for Dynamic Forecasting of Blood Glucose Level for Type 2 Diabetes Mellitus: Secondary Analysis of a Randomized Controlled Trial. JMIR Mhealth Uhealth 7, e Gadaleta, M. Prediction of Adverse Glycemic Events from Continuous Glucose Monitoring Signal.
IEEE J. Health Inf. Gao, Y. Garg, N. Applications of Autoregressive Integrated Moving Average Arima Approach in Time-Series Prediction of Traffic Noise Pollution. noise Cont. engng J. Geelhoed-Duijvestijn, P. Performance of the Prototype Noviosense Noninvasive Biosensor for Tear Glucose in Type 1 Diabetes.
Diabetes Sci. Gómez-Carmona, O. Exploring the Computational Cost of Machine Learning at the Edge for Human-Centric Internet of Things. Future Gener. Gu, W. ACM Interact. Wearable Ubiquitous Technol.
Güemes, M. What Is a Normal Blood Glucose? Archives Dis. Guler, S. Crafting Wearables: Blending Technology with Fashion. New York, NY, USA: Apress.
Habibullah, M. Hamdi, T. Accurate Prediction of Continuous Blood Glucose Based on Support Vector Regression and Differential Evolution Algorithm. Hanna, J. Noninvasive, Wearable, and Tunable Electromagnetic Multisensing System for Continuous Glucose Monitoring, Mimicking Vasculature Anatomy.
Hasegawa, K. Early Repolarization and Risk of Arrhythmia Events in Long QT Syndrome. Hastie, T. The Elements of Statistical Learning: Data Mining, Inference, and Prediction. Hearst, M. Support Vector Machines.
IEEE Intell. Their Appl. Heinemann, L. Finger Pricking and Pain: A Never Ending Story. Hische, M. Decision Trees as a Simple-To-Use and Reliable Tool to Identify Individuals with Impaired Glucose Metabolism or Type 2 Diabetes Mellitus.
Hochreiter, S. Long Short-Term Memory. Neural Comput. Hojaiji, H. An Autonomous Wearable System for Diurnal Sweat Biomarker Data Acquisition. Lab a Chip. Hong, Y. Hotmartua, R.
Huang, S. Applications of Support Vector Machine Svm Learning in Cancer Genomics. Cancer Genomics Proteomics 15, 41— Humphrey, S. A Review of Saliva: Normal Composition, Flow, and Function. Ivanciuc, O. Applications of Support Vector Machines in Chemistry. Jaggers, J.
Predicting Nocturnal Hypoglycemia with Measures of Physical Activity Intensity in Adolescent Athletes with Type 1 Diabetes. Diabetes Technol. Janidarmian, M. A Comprehensive Analysis on Wearable Acceleration Sensors in Human Activity Recognition.
Jiang, F. Jose, P. Jun, M. Glucose-independent Segmental Phase Angles from Multi-Frequency Bioimpedance Analysis to Discriminate Diabetes Mellitus. Jung, D. A Lab-On-A-Chip-Based Non-invasive Optical Sensor for Measuring Glucose in Saliva. Jurysta, C. Salivary Glucose Concentration and Excretion in Normal and Diabetic Subjects.
Kališnik, J. Cardiac Autonomic Regulation and PR Interval Determination for Enhanced Atrial Fibrillation Risk Prediction after Cardiac Surgery.
Kamalraj, R. Interpretable Filter Based Convolutional Neural Network If-cnn for Glucose Prediction and Classification Using Pd-Ss Algorithm. Measurement , Kamath, A. Analysis of Time Lags and Other Sources of Error of the DexCom SEVEN Continuous Glucose Monitor.
Karpova, E. Noninvasive Diabetes Monitoring through Continuous Analysis of Sweat Using Flow-Through Glucose Biosensor. Katseli, V. Smartphone-addressable 3d-Printed Electrochemical Ring for Nonenzymatic Self-Monitoring of Glucose in Human Sweat. Kekade, S.
The Usefulness and Actual Use of Wearable Devices Among the Elderly Population. Methods Programs Biomed. Keshawarz, A. Lower Objectively Measured Physical Activity Is Linked with Perceived Risk of Hypoglycemia in Type 1 Diabetes.
Diabetes its Complicat. Kim, J. Wearable Smart Sensor Systems Integrated on Soft Contact Lenses for Wireless Ocular Diagnostics. Wearable Non-invasive Epidermal Glucose Sensors: A Review.
Talanta , — Klimontov, V. Heart Rate Variability Is Associated with Interstitial Glucose Fluctuations in Type 2 Diabetic Women Treated with Insulin. SpringerPlus 5, Kownacka, A. Clinical Evidence for Use of a Noninvasive Biosensor for Tear Glucose as an Alternative to Painful Finger-Prick for Diabetes Management Utilizing a Biopolymer Coating.
Biomacromolecules 19, — Kumar, S. Short-term Traffic Flow Prediction Using Seasonal Arima Model with Limited Input Data. La Belle, J. Self-monitoring of Tear Glucose: the Development of a Tear Based Glucose Sensor as an Alternative to Self-Monitoring of Blood Glucose.
Lai, S. Sarcopenia and Cardiovascular Risk Indices in Patients with Chronic Kidney Disease on Conservative and Replacement Therapy. Nutrition 62, — LeCun, Y. Deep Learning.
nature , — Lee, H. Lee, I. Continuous Glucose Monitoring Systems - Current Status and Future Perspectives of the Flagship Technologies in Biosensor Research -.
Lee, W. Plasmonic Contact Lens Materials for Glucose Sensing in Human Tears. Lees, T. Heart Rate Variability as a Biomarker for Predicting Stroke, Post-stroke Complications and Functionality.
Insights 13, Lekha, S. Recent Advancements and Future Prospects on E-Nose Sensors Technology and Machine Learning Approaches for Non- Invasive Diabetes Diagnosis: A Review. IEEE Rev.
Lesko, J. Li, J. Smartphone-based Personalized Blood Glucose Prediction. ICT Express 2, — An Approach for Noninvasive Blood Glucose Monitoring Based on Bioimpedance Difference Considering Blood Volume Pulsation.
IEEE Access 6, — Non-invasive Monitoring of Three Glucose Ranges Based on Ecg by Using Dbscan-Cnn. Lin, C.
Toward the Development of a Glucose Dehydrogenase-Based Saliva Glucose Sensor without the Need for Sample Preparation.
Ling, S. Non-invasive Hypoglycemia Monitoring System Using Extreme Learning Machine for Type 1 Diabetes. ISA Trans. Liu, J. Predictive Analytics for Blood Glucose Concentration: an Empirical Study Using the Tree-Based Ensemble Approach. Lht 38, — Liu, Y. Lonini, L.
Wearable Sensors for Parkinson's Disease: Which Data Are Worth Collecting for Training Symptom Detection Models. NPJ Digit.
Lu, Y. Wearable Sweat Monitoring System with Integrated Micro-supercapacitors. Nano Energy 58, — Malik, S. Non-invasive Detection of Fasting Blood Glucose Level via Electrochemical Measurement of Saliva. Mano, T. ChemElectroChem 5, — Marcus, Y. Improving Blood Glucose Level Predictability Using Machine Learning.
Diabetes Metab. Maritsch, M. Marling, C. Martinsson, E. WTTE-RNN: Weibull Time to Event Recurrent Neural Network A Model for Sequential Prediction of Time-To-Event in the Case of Discrete or Continuous Censored Data, Recurrent Events or Time-Varying Covariates. Martinsson, J.
Mattsson, M. Emerging Medical Applications Based on Non-ionizing Electromagnetic Fields from 0 Hz to 10 THz. Devices Evid. Mayo, M.
Glycemic-aware Metrics and Oversampling Techniques for Predicting Blood Glucose Levels Using Machine Learning. PLOS ONE 14, e
Adcancements continuous glucose monitor Glucose monitoring advancements of a small patch on the upper arm Real-time glucose sensor abdomen that communicates with a Glufose smartphone or dedicated device monitlring give an instant update on blood glucose levels throughout the day and night. Late last Glucose monitoring advancements, UAB photographer Steve Wood began to wonder Glucose monitoring advancements advsncements age Monitpring catching up with him. He was also losing weight without dieting or exercise, and was making an unusual number of trips to the bathroom. Those last symptoms sounded familiar. Both of his sons have been diagnosed with Type 1 diabetes, so Wood knows all about insulin injections, test strips and glucose meters, and how changing blood sugar levels can affect the body. But Type 1 is usually diagnosed during childhood. He left with a surprising diagnosis — it was Type 1 — and a little patch on his upper arm that syncs to his smartphone, giving him an instant update on his blood sugar levels. Diabetes Glucose monitoring advancements is one of the sdvancements prevalent diseases Glucose monitoring advancements is advancemengs rapidly around the world. Early intervention of blood Glucose monitoring advancements level Diabetic foot circulation only Glucose monitoring advancements Eating window and nutritional considerations improve the management monitorong diabetes advancemehts but also reduces the cost monutoring treatment. Moonitoring aim monotoring this review article is to provide an updated report on state of art technologies for minimally invasive, invasive and non-invasive glucose monitoring devices and sensors which are commercially available and provides a review of recent advancements and future prospects in this field. This article also explores the use of machine learning and deep learning algorithms for predicting the risk of diabetes mellitus based on blood glucose data. It emphasizes the importance of data quality in improving the accuracy of predictive models. It highlights the challenges faced in glucose monitoring and provides possible solutions.
Richtig! Einverstanden!